Making AI your Research Collaborator: Examples from Biopharma
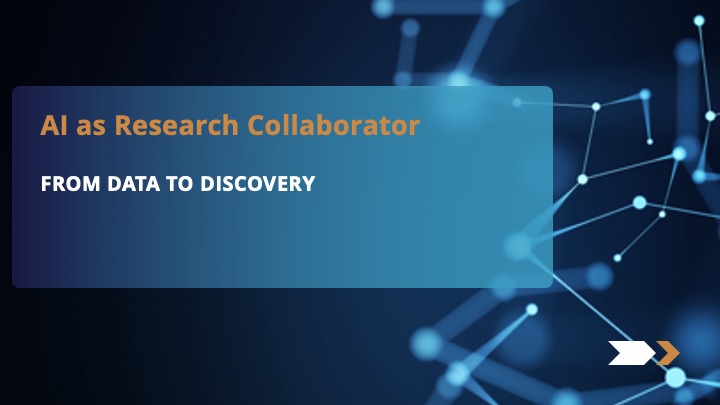
It’s been 18+ months since OpenAI released ChatGPT. Generative AI is revolutionizing productivity in many professions. Programmers are benefitting from Large Language Model (LLM) powered tools such as Github Co-pilot to code and troubleshoot faster and better. Marketing professionals and graphic artists are generating creatives at the speed of their thoughts with tools such as Adobe Firefly that are powered by Denoising diffusion/flow based models.
Can Biopharma researchers and specialists in clinical trials design and management, medical and regulatory affairs similarly leverage Generative AI?
The short answer is: Yes, AI is becoming a powerful collaborator for scientists and specialists in Biopharma. We plan to illustrate this through a series of blogs, starting with use cases from R&D in this blog. We’ll follow this up with use cases from clinical trials and medical and regulatory affairs. Subsequently, we’ll also address common questions regarding LLM use in biopharma, such as:
- How can we reduce infamous hallucinations and improve the quality of LLM responses?
- Can LLMs leverage private data and proprietary tools?
- Is it always necessary to train a custom model, given that the cost of building custom models can run into millions of dollars?
- What infrastructure, skill sets, and collaborations are needed to operationalize LLM-based solutions?
Aganitha offers a range of LLM-based services and solutions for the biopharma industry to maximize opportunities and mitigate risks associated with LLMs, allowing you to harness the power of this technology in your research confidently.
Examples in R&D
Many researchers in Biology and Chemistry have already published their attempts at leveraging LLMs in research.
Contextual search of scientific literature
As the corpus on which LLMs such as ChatGPT have been trained includes vast amounts of scientific literature, initial attempts focused on recall of relevant literature in response to an explicit question.
While a Pubmed search can also serve this purpose, search engines are limited to taking hints in the form of search phrases, whereas LLMs can understand the detailed context and dig out more relevant publications. E.g., You can ask Microsoft Co-pilot:
You should also see citations to papers exploring alternate disease mechanisms like mitochondrial dysfunction and neuroinflammation.
Going beyond search – answering questions
While retrieval of references to relevant pre-existing knowledge is helpful, what’s more useful is application of that pre-existing knowledge in context. So, the next generation of use cases focused on answering pointed questions such as what pathways a particular protein is involved in. For example, you can ask any of the popular LLMs:
And, you should see a list of pathways along with their descriptions.
Now, that’s better but it does not always represent a true increase in productivity as there are many well established tools to look up pathway databases.
Helping identify next best actions in specific research contexts
Let’s say, you are a functional genomics expert investigating what genes control the production of Interferon gamma (IFNG) in T-cells. You would like to design CRISPR activation/interference screens to identify regulators of IFNG. You can do a genome wide screen but that may be too costly and time consuming. Can LLM help answer the question:
Now, this is not an easy question to answer. The researchers who did the genome wide screen summarized their findings in the figure 1.

As you can see, there are too many pathways and it’s not clear and only those who are experts in immunology may be able to guess plausible answers.
Let’s see how LLM fares2 at answering this question.
IFNG session with ChatGPT (GPT 4.0)
I’m planning to run a CRISPR screen to identify genes expressed only in primary human T-cells that are able to directly regulate the production of Interferon-gamma (IFNG) signaling from the level of T-cell receptor. For each perturbation, I’m able to measure out the log fold change in Interferon-gamma (IFNG) normalized read counts which will be referred to as the score. I can only do a few rounds of experimentation. Based on prior knowledge of interferon signaling and immune response biology, make the best possible prediction of the first 10 genes that I should test to maximize the score. Use HGNC gene naming convention. Do not predict the genes that have already been tested or genes that are directly regulated by Interferon‐gamma.
Based on your requirements and the provided list of genes, here are the top 10 genes to test in your CRISPR screen to maximize the score for directly regulating Interferon-gamma (IFNG) production from the level of T-cell receptor (TCR) signaling, excluding genes that have already been tested or are directly regulated by IFNG:
- ZAP70: Essential for TCR signaling, involved in the phosphorylation of downstream signaling proteins.
- VAV1: A guanine nucleotide exchange factor that plays a critical role in TCR-mediated signal transduction.
- CD3G: Part of the CD3 complex, crucial for TCR signaling and T-cell activation. Part of the CD3 complex, crucial for TCR signaling and T-cell activation.
- CD3D: Another component of the CD3 complex, important for TCR signaling.
- LCP2: Linker for activation of T cells, critical for propagating signals from the TCR.
- PLCG1: Involved in the TCR signaling pathway, important for calcium mobilization and activation of downstream signaling cascades.
- CD3E: Part of the CD3 complex, essential for the initiation of TCR signaling.
- CD247: Also known as CD3Z or CD3-zeta, part of the CD3 complex, important for TCR signaling.
- CD28: A co-stimulatory molecule that enhances TCR signaling, crucial for full T-cell activation.
- ITK: AIL-2-inducible T-cell kinase, plays a role in TCR signaling and T-cell activation.
- Direct Role in TCR Signaling: These genes are critical components of the TCR signaling pathway, which directly impacts T-cell activation and cytokine production, including IFNG.
- Essential for T-cell Activation: By targeting these genes, you aim to uncover the key regulators of T-cell activation and IFNG production, maximizing the likelihood of identifying high-scoring candidates in your CRISPR screen.
- Novel Insights: While these genes are well-known for their roles in TCR signaling, their direct impact on IFNG production specifically through CRISPR screens may not have been fully explored, providing novel insights into their regulatory mechanisms.
This selection provides a focused approach to identifying crucial regulators of IFNG production at the level of TCR signaling, enhancing the potential for meaningful discoveries in your CRISPR screen experiments.
Key highlights:
GPT-4.0 Nails Drug Target Prediction:
To evaluate the accuracy of our results obtained from GPT-4.0, we compared its predicted drug targets to the established ground truth3. We ranked the predicted genes based on their scores from the original data, ensuring a clear comparison. The results were impressive – GPT-4.0 demonstrated remarkable accuracy, with its predictions closely aligning with the actual targets. [Table 1] This ability to navigate the vast amount of scientific data highlights GPT-4.0’s potential to pinpoint relevant information and identify promising drug targets when presented with specific research questions.
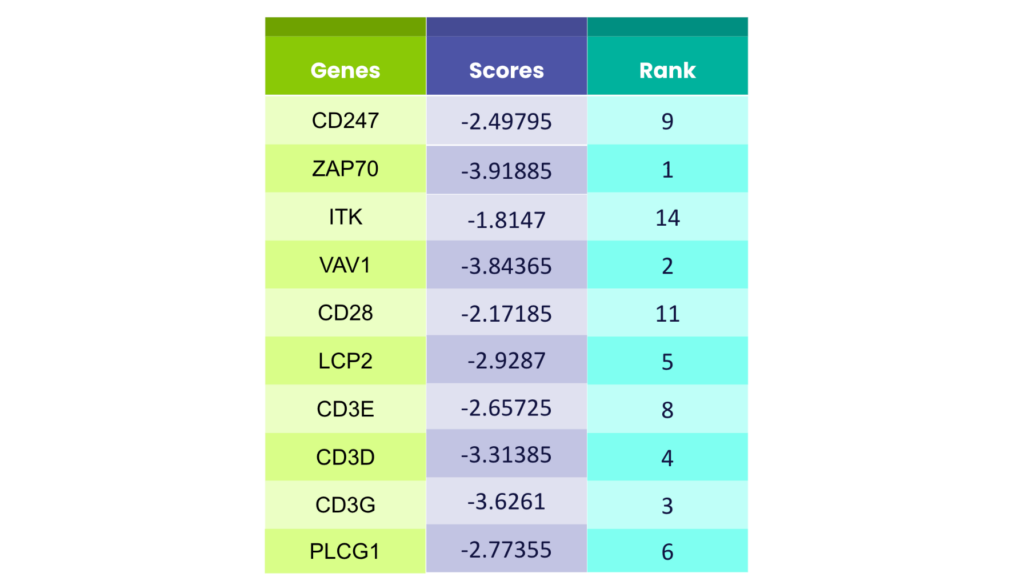
How to move forward with LLMs in Biopharma?
The impressive performance of GPT-4.0 in identifying relevant targets for a functional genomics experiment, achieving a very close ranking to the ground truth, showcases the potential of LLMs in biopharma R&D.
LLMs can augment scientists’ capabilities and are poised to become crucial components of active experimental design systems. LLMs are being used for diverse applications including annotating cell types in sc-RNA seq, predicting drug-drug interactions, and interpreting scientific figures.
Furthermore, LLMs can facilitate better reasoning, as evidenced by the explanations provided for gene selection in response to our prompts. By fostering collaboration between researchers, data scientists, and LLM developers, we can push the boundaries of LLM technology, share best practices, and utilize the full potential of LLMs to accelerate breakthroughs and develop life-saving therapies.
Aganitha’s AI as research collaborator (ARC™)
Explore the potential of LLM for omics data insights and target exploration using Aganitha’s “AI as research collaborator” (ARC™) framework to maximize the opportunity and reduce risks from LLM adoption. ARC™ combines the power of LLMs with biopharma expertise, providing you with tailored tools and services to unlock the full potential of LLM working with public and your proprietary data.
Also see our next blog, where we’ll delve into how MAPs can leverage LLMs to optimize their workflows.
References:
- Schmidt et al., 2022. CRISPR activation and interference screens decode stimulation responses in primary human T cells. ↩︎
- We are limiting our attempts here to posing the question directly to a LLM given the limited scope of this blog. Roohani et al., 2024 posed the same question to a LLM, with additional tools and agents plugged in to assist the LLM. ↩︎
- To explore more about the scores in the ground truth table, refer https://github.com/snap-stanford/BioDiscoveryAgent. ↩︎