Leveraging AI for protein fitness optimization
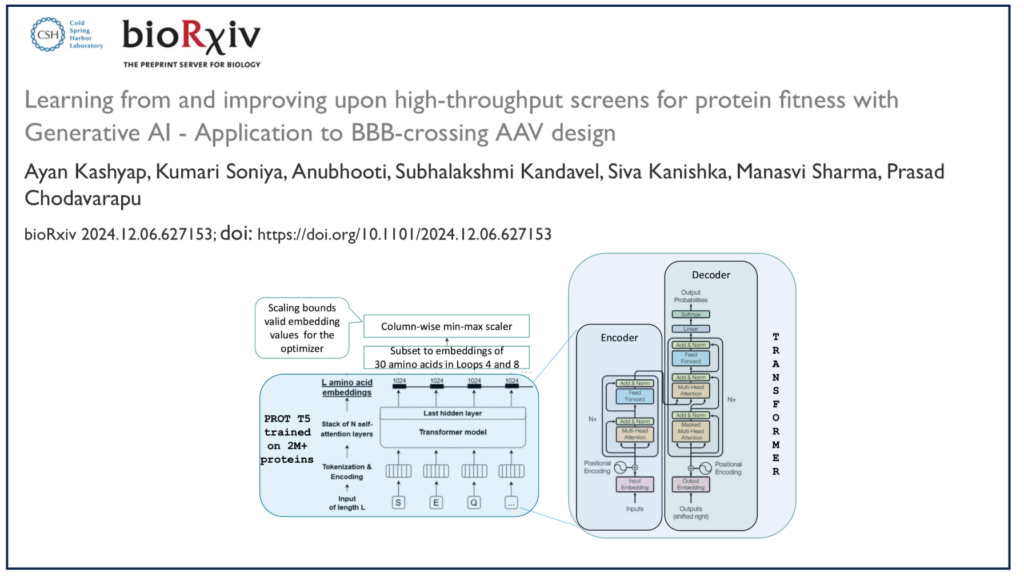
AI has emerged as a transformative force in solving complex biological challenges in the era of data-driven innovation. At the intersection of biotechnology and computational science, AI is reshaping how we approach the design and optimization of biological entities such as proteins.
The challenge of protein fitness optimization
Designing proteins with desirable traits involves exploring a vast sequence space. For example, optimizing Adeno-Associated Virus (AAV) capsid variants for tropism using directed evolution (DE) methods like M-CREATE and TRACER tests only 0.04% of the 2.8 trillion possible nucleotide sequences. Despite state-of-the-art high-throughput techniques, much of the design space remains unexplored, requiring reinforcement to navigate its complexity—this is where AI steps in.
Why protein fitness optimization matters
Beyond optimizing viral vectors, protein fitness optimization can be used to improve enzyme catalytic efficiency and stereoselectivity, develop stable antibodies, and design novel therapeutic modalities. It is also useful for optimizing enzymes for activity, expression, stability, and solubility.
AutoMaxProFit: A Generative AI approach to protein design
In a recent preprint, our team at Aganitha disclosed a generative AI-based method, Autograd based maximization of protein fitness (AutoMaxProFit), that leverages protein language models (pLMs) and transformer-based architectures to learn from directed evolution experiment results and optimize protein sequences for desired function.
A Direct AI-Driven Approach
AutoMaxProFit introduces a systematic method to fine-tune protein designs:
Data Utilization: Leveraging Directed Evolution (DE) generated data, we fine-tuned a transformer-based protein language model to predict fitness scores.
Gradient Optimization: Using autograd-enabled gradient ascent, we explored the embedding space to identify optimal protein sequence embeddings.
Decoding Sequences: The optimized embeddings were mapped back into amino acid sequences using a de-masking trick.
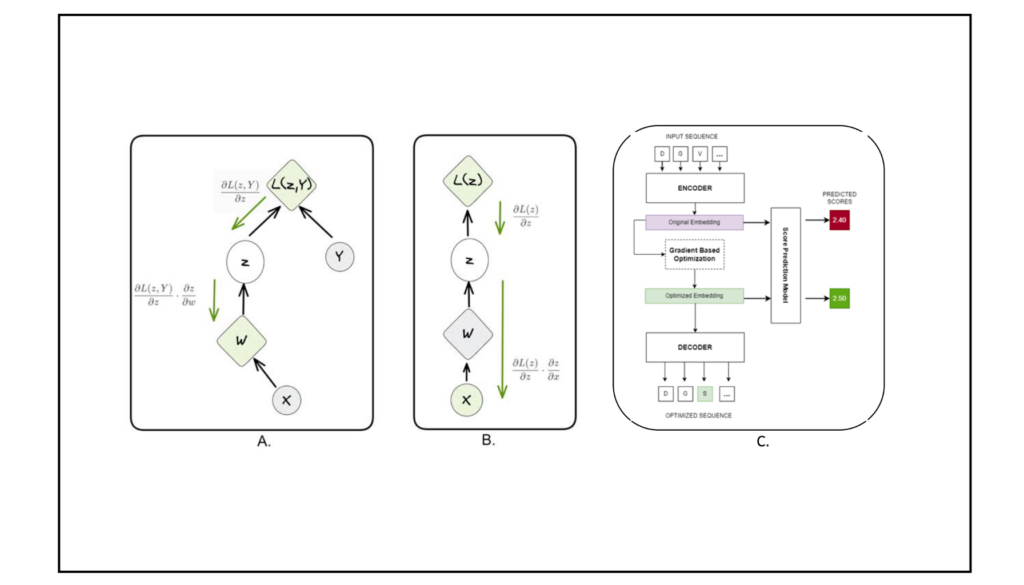
Fine-Tuned Loss Function
The optimizer’s loss function combined predicted fitness scores with a penalty for straying outside the embedding sub-space covered by the training dataset. This ensured the generated sequences remained biologically plausible while maximizing fitness predictions. [Figure 2]
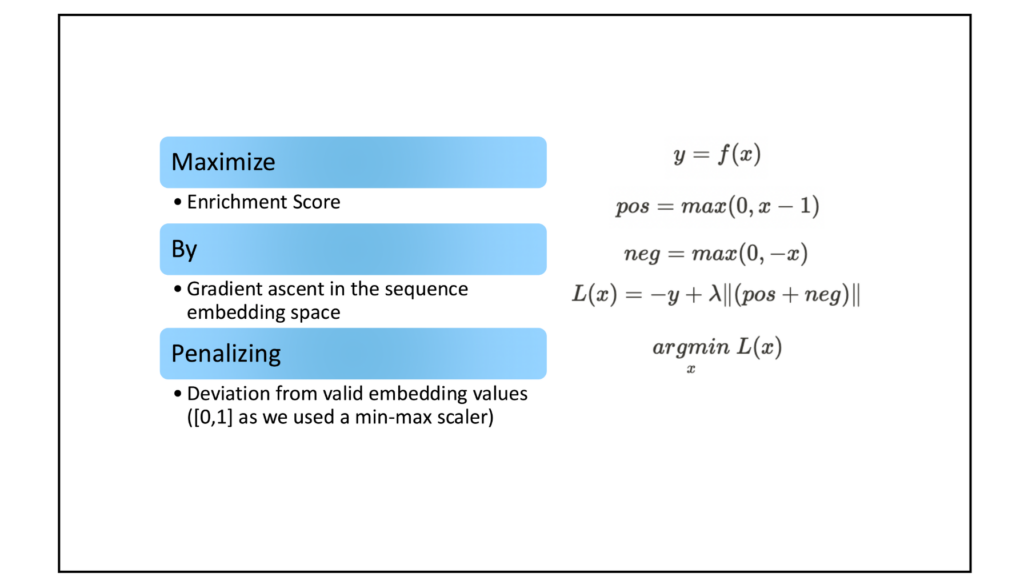
Deep learning meets biopharma: A case study with AAV9
To showcase AutoMaxProFit’s capabilities, we optimized an AAV9 capsid sequence for blood-brain barrier (BBB) penetration. This approach yielded a 4x improvement in binding to LY6A receptors, targets of BBB-penetrant variants in mice models used by DE experiments.
Key findings: Changes made by AutoMaxProFit
Table 1 demonstrates the power of AutoMaxProFit in optimizing AAV capsid sequences. Starting with the sequence “TLQLPFK“, a top-performing 7-mer insert identified in the M-CREATE experiment, AutoMaxProFit generated variants with higher predicted enrichment scores. Enrichment score represents the relative success of a capsid sequence in penetrating a target tissue or cell type. A higher enrichment score indicates a greater ability of the AAV variant to reach the desired cells. The sequence “SLQALQA” exhibited the highest predicted enrichment score (3.39), suggesting it might be the most effective at targeting brain endothelial cells. The progression of sequence changes illustrates the optimizer’s ability to refine designs to achieve desired traits. [Table 1]

Technical validation via MD simulations
Molecular Dynamics (MD) simulations validated these results by quantifying binding interactions between the optimized capsid and LY6A receptors. [Figure 3]
Structural Insights: The LY6A receptor is extensively engaged with the trimeric AAV9 capsid protein for BBB-penetrant capsids. In contrast, interactions were limited with the trimer with lesser buried surface area and binding affinity for non-penetrants.
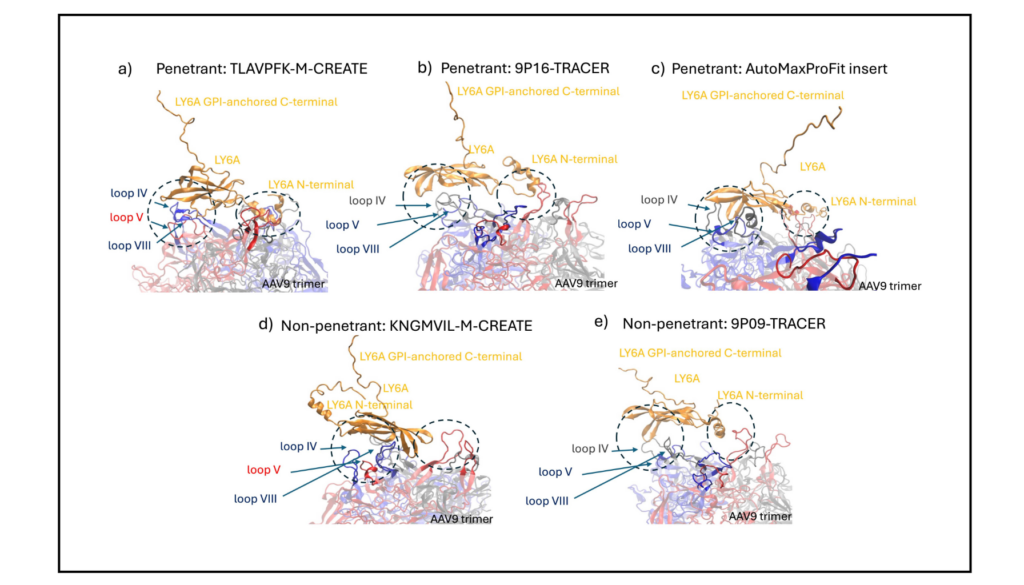
Expanding the horizons of AI in biopharma
The goal of protein fitness opt keeps occurring in multiple contexts in biopharma design. For example: Engineering enzymes with improved catalytic efficiency, substrate selectivity and product specificity. We will be talking about these in subsequent blogs. As AI continues to integrate deeper into biotechnology, frameworks like AutoMaxProFit exemplify the synergy between data science and biological discovery. By combining transformer-based architectures with gradient optimization and molecular simulations, we are unlocking new possibilities in protein design.
Dive deeper
AutoMaxProFit is more than a tool—it’s a glimpse into the future of protein engineering. To dive deeper into the technical details and the groundbreaking methodologies behind this innovation, read our full preprint here.
To learn more about our work, visit our Gene and Cell Therapies Solutions page.