Accelerating antibody & nanobody design for research use cases with Generative AI
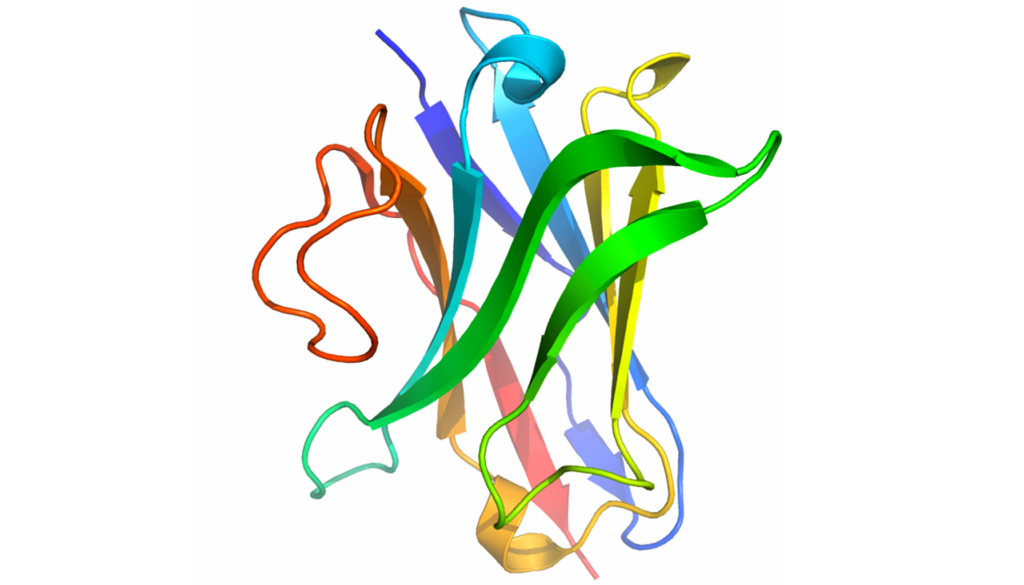
Role of Antibodies in research
Antibodies are foundational tools in research and diagnostics in addition to being the largest biological modality in therapeutics. Antibodies are highly useful reagents in research due to high specificity and selectivity; to detect, quantify and purify proteins/peptides.
Challenges of Traditional Antibody Discovery for research use cases
Unfortunately, reliability of research antibodies has been a persistent issue. False positives and negatives can lead to wasted time, effort, and even damage a researcher’s reputation. There are many sources for reliability problems in research antibodies. One key source of trouble came from use of immunized animals (such as rabbits) for discovery and production of research antibodies. It meant high costs and long cycle times in discovery and batch-to-batch variability in production. Move to recombinant approaches for antibody production, along with establishment of validation standards have helped address challenges in production. But challenges in discovery remain.
Phage display provides an alternative discovery path but it requires large diverse libraries of antibodies which are capable of producing hits against a variety of novel targets researchers study. Hence, rabbits continued to be relied upon for generation of research antibodies. Rabbits have a more diverse immune repertoire than mice and are more distant to humans than mice, allowing them to produce antibodies against targets that may be considered self in mice. To summarize, the challenge of generating antibodies against hard to target antigens in addition to the challenges of longer time cycles and of non-specific antibodies still persist.
This raises a crucial question: wouldn’t it be better if we are able to design antibodies for a novel target using computational methods, eliminating the need for rabbits and reducing costly/time-consuming in vitro and in vivo experimentation?
Generative AI: Revolutionizing Antibody Design
The emergence of Generative AI and large language models in biology has opened up exciting possibilities for antibody design. This technology allows for:
De novo design:
Generative AI can design antibodies in silico , eliminating the need for animals and reducing reliance on existing libraries.
Faster discovery:
Generative AI can generate a vast number of candidate antibody designs in a short period.
Aganitha’s Generative AI Platform for Antibody Design
We at Aganitha have been early adopters of Generative AI based approaches for de novo design and have developed platforms for both small molecule and antibody design. Our antibody design solution combines Generative AI methods and Protein language models with Molecular Dynamics (MD) and other physics-based approaches to address complex analysis and validation needs.
Nanobodies are designed for tailored applications:
Use cases requiring smaller and simpler designs led to discovery and adoption of nanobodies and scFVs. Nanobodies offer several advantages over conventional antibodies in research, diagnostic as well as therapeutic applications. Derived from camelids, nanobodies consist of a variable heavy-chain domain (VHH) weighing around 15kDa and have structural and functional advantages that make an attractive candidate in many use cases.
In response to demand for Nanobody design, we at Aganitha have enhanced our antibody design pipeline to generate nanobodies as well. Working with CCMB, we are applying our pipeline to generate nanobody targeting a class of neurotransmitter receptors that are of interest in research of CNS disorders (Figure 1).
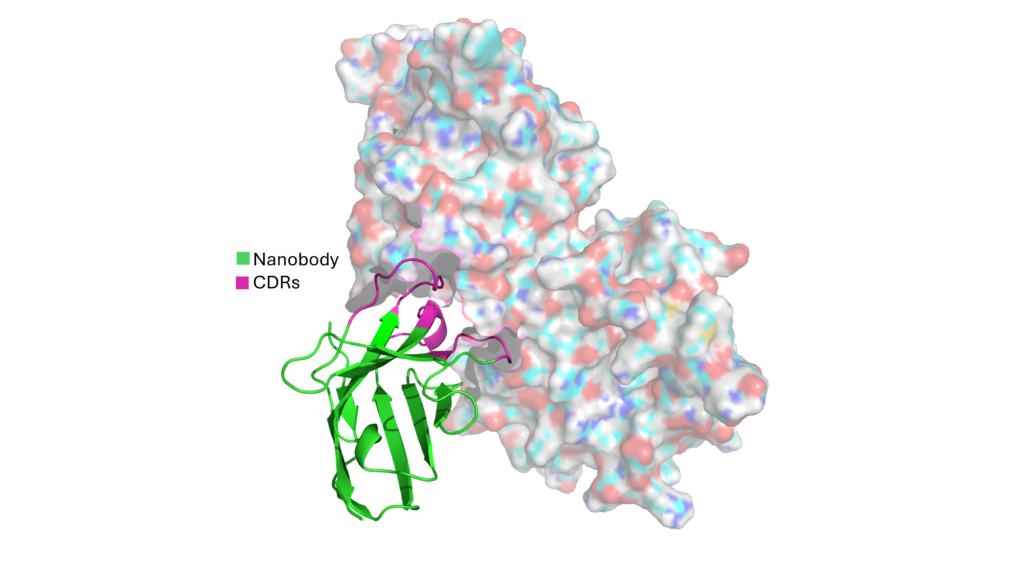
Challenges of In Vitro Validation
In silico approaches for antibody/nanobody design can produce hundreds to thousands of candidate designs at the press of a button. While the designed candidates can be and indeed are validated using in silico methods, validating them all in vitro remains a time-consuming and expensive bottleneck. High-throughput robotic experimentation does not completely address this challenge.
The Future of Antibody Discovery with Generative AI requires better unit economics for validation
The future of antibody discovery lies in developing cost-effective validation protocols. These protocols should involve:
- Minimal DNA synthesis: Reducing the amount of DNA synthesis required for each candidate.
- Simplified cloning: Streamlining the process of cloning candidate designs into vectors.
- Robust and inexpensive expression systems: Developing reliable and cost-effective methods for expressing the designed antibodies.
- High-throughput validation systems: Implementing efficient systems for validating a large number of candidates simultaneously.
By achieving these advancements, Generative AI has the potential to revolutionize antibody discovery, leading to a faster and more efficient process for researchers.